posted 09-10-2008 11:53 PM
I'll argue, in principle, that it is unethical to acquiesce, abandon, or surrender one's professional judgment to any test or computer score. Tests don't make decisions, they give information. Decision are made by professionals, who should be capable of explaining and arguing the merits of their decisions. I'll also argue that professionals have an ethical obligation to use the best and most powerful tools available when gathering and evaluating the information on which they base their decisions.
Computers can, in theory, outperform humans in some data evaluation tasks because they can execute complex math and procedures quickly and with perfect reliability. My computer doesn't care if my examinee smells bad, or if I have a headache.
However, there is no credible argument that our present computer scoring algorithms would outperform humans with uninterpretable data. It might be possible to develop algorithms to automate the detection of artifacted or uninterpretable segments of data. Keep in mind, however, that to a computer the detection of an artifact is really just another data problem – in which the artifact itself is the data of interest, with two questions of concern: 1) is it actually an artifact or un-scorable segment, and 2) how did it come to occur when it did (i.e., by random chance or strategic effort).
OSS-3, for both Lafayette and Limestone, includes a special equation to calculate the statistical probability that artifacted and uninterpretable segments have occurred by random chance alone. So, we have an emerging solution for the 2nd question. The first question is what we call “non-trivial,” and human pattern recognition approaches are presently superior to what computers have been trained to do. It will be interesting to see what the future holds.
Computers should out-perform humans with normal data, primarily because they can execute more aggressive decision policies (rules and alpha boundaries), while using statistical models to constrain errors and inconclusives. Human examiners will not likely take the time to work on a bunch of advanced math while preparing to interrogate an examinee. Human scoring models are simplistic additive models, which constrain errors through cautious decision rules, and cautious cutscores (which are really just unknown alpha boundaries). These simplistics mathematics are reflected in polygraph experience and polygraph research.
Consider the venerable MGQT, in your favorite flavor. Imagine 4 RQs in some police pre-employment situation, involving targets such as involvement with drugs, history of violence, other crimes, and whatever other investigation targets are actuarially related to police training success and police job performance success. Now imagine a 25% base-rate for each of those four issues. Which are really four separate, but simultaneous, tests. Also imagine the issues are independent of each other. You can estimate the proportion of truthful examinees as the product of the inverse of the four base-rates (.75 x .75 x .75 x .75 = .32). We use the multiplication-rule for combining independent probability events. Yep, only about 32% of examinees can be expected to be truthful to all four targets, if the base rates are 25% per each. Then consider at test for which we'll assume the sensitivity rate is 90% (.9), with false positives at 5% (.05) and inconclusives at 5% (.05). Some would say these are optimistic numbers. In field practice, any DI result means a DI test, so your sensitivity rate is not depleted by the combinatoric problems, and remains at .9. What is depleted is the power of the test to discriminate which question the examinee lied to. For that we would estimate the reduction in discrimination by multiplying .9 for each of the four target questions, (.9 x .9 x .9 x .9 = .66). The more questions you add, the greater the likelihood that you fail to catch something, and the less capable the test will be at telling you which issue to interrogate on. Of course, you can always interrogate on everything, but your examinee may know when you are focused and when you are un-focused. This is part of why I am opposed to the five targets, as described in the APA model policy on screening exams. Its trying to do too much.
Now look at the truthful side of the problem. Assume the MGQT has a specificity rate of 90% (.9), but we have to combine that for each of the four questions. So, the independent probability that we get a correct truthful score to each of four truthful questions (for the 32% of police applicants who are expected to be truthful to all four investigation targets) is (.9 x .9 x .9 x .9 = .66), with the dependent probability of an inconclusive result at (.05 + .05 + .05 + .05 = .2) and the dependent probability of a FP error at (.05 + .05 + .05 + .05 = .2). INCs and FPs are non-independent, because any INC or error means the test is an INC or error.
Its no surprise that studies have shown the MGQT to provide good sensitivity to deception and weaker performance with truthful subjects. What we may not have thoroughly considered is that the problem with the MGQT may not be with the test structure, but with out scoring procedures and simplistic/additive decision models.
BTW, I didn't make this up, and I didn't just figure this out one day. This is a set of problems known to statisticians and researchers everywhere. Its called “multiple comparisons.” We often discuss this problem in terms of “inflated alpha.” There are known and well studied procedural and mathematical solutions to this phenomena. We simply have to learn about them and talk more about them (and we have to learn to not be afraid of advanced statistics).
Senter (2003) studied decision rules for MGQT exams, and suggested that two-stage rules may help. My guess is that he is well aware of these complications, and limited his solutions to procedural/rule solutions due to interest in solutions that can be easily incorporated by field examiners who hand score their tests. In my view, two-stage rules are a procedural approximation or procedural solution to this same problem of multiple comparisons and inflated alpha.
The common statistical solutions to multiple comparison and inflated alpha problems are are to make sure we understand the location and shape of the distributions of our data, and to make strategic use of omnibus (everything at once) statistical models such as ANOVAs, and post-hoc tests (such as Tukey's or Bonferonni) of individual issues (sounds a little like a break-out test, doesn't it). These advanced mathematical models can be used to score tests aggressively and reduce inconclusives while still constraining errors. Of course, errors will always occur – its just that we only hear about errors at certain times.
Another possible solution to improved scoring might be to more assertively simplify our scoring systems, retaining only the most robust proven ideas. Think about this: computer scoring algorithms have consistently employed simpler (not more complex) physiological features than human scoring systems, and they have consistently employed more structured operational procedures than human scoring systems. Computers cannot make subjective decisions, they simply do what they are instructed to do. Even AI system are not truly subjective, but employ a structured rule or principle to make a pseudo-subjective decision.
You'll understand the challenge if you just try to make a decision tree to display every possible choice or decision a human makes when scoring a test. Along the way, you'll have to make every decision with a mathematical equation, Without measurement and without math, all you'll have is a sorting procedure. Sorting procedures lack sound mathematical theory, are limited to simplistic frequency methods for empirical support, and cannot provide an inferential or probability estimate. Then, structure your model so it can adapt to and accommodate the entire range of polygraph techniques and practices while meeting the requirement for a completely logical decision tree. Most hand-scoring systems won't meet this challenge. Solve the problem and you'll have a complex decision model, which could also programmed into a computer scoring algorithm.
The real point of all this if we want to improve the polygraph test we should become more willing to harness the power of our computers to do the coma-inducing math for us. That doesn't mean let the computer score the test. It means let the computer assist us in scoring the test.
If we're going to use a computer algorithm to help score or help QC our charts, we still have some ethical and professional obligations. It is still the examiner who has to administer a proper test, with proper questions, regarding a proper testable issue. It is still the examiner who has to ensure that the data scored by the computer is reasonable and proper interpretable data. Another ethical obligation is that we have the ability to understand what goes on inside the computer algorithm. Field examiners should not have to know how to actually calculate every statistical formula to aggressively score a polygraph test. However, it might be reasonable to expect field examiners to know more about the statistical models that can improve polygraph science. It might also be reasonable to expect that field examiners and researchers have the ability to know what exactly an algorithm does. This is a lot easier than it sounds. All that is required is complete documentation of the algorithm's procedures. We should be able to sit down with a calculator and note-pad (as if we have excess time to kill) and calculate the same test result as the algorithm. Not that we would ever want to actually do so, but we should be able to do so if we wanted to or had to. Its the same reason we keep certain text books from college and graduate school.
Computers still depend on good data or the scores become meaningless, just as human hand-scores become meaningless when the data stink. Remember the old computer adage: garbage in = garbage out. A rule of thumb should be: if you wouldn't score it, then you shouldn't let the computer score it. The important question then becomes: why is the data un-scorable.
In the end, it is the examiner who has to render an opinion about truthfulness or deception. But why not let the computer assist us with the powerful math?
r
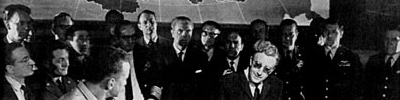
------------------
"Gentlemen, you can't fight in here. This is the war room."
--(Stanley Kubrick/Peter Sellers - Dr. Strangelove, 1964)